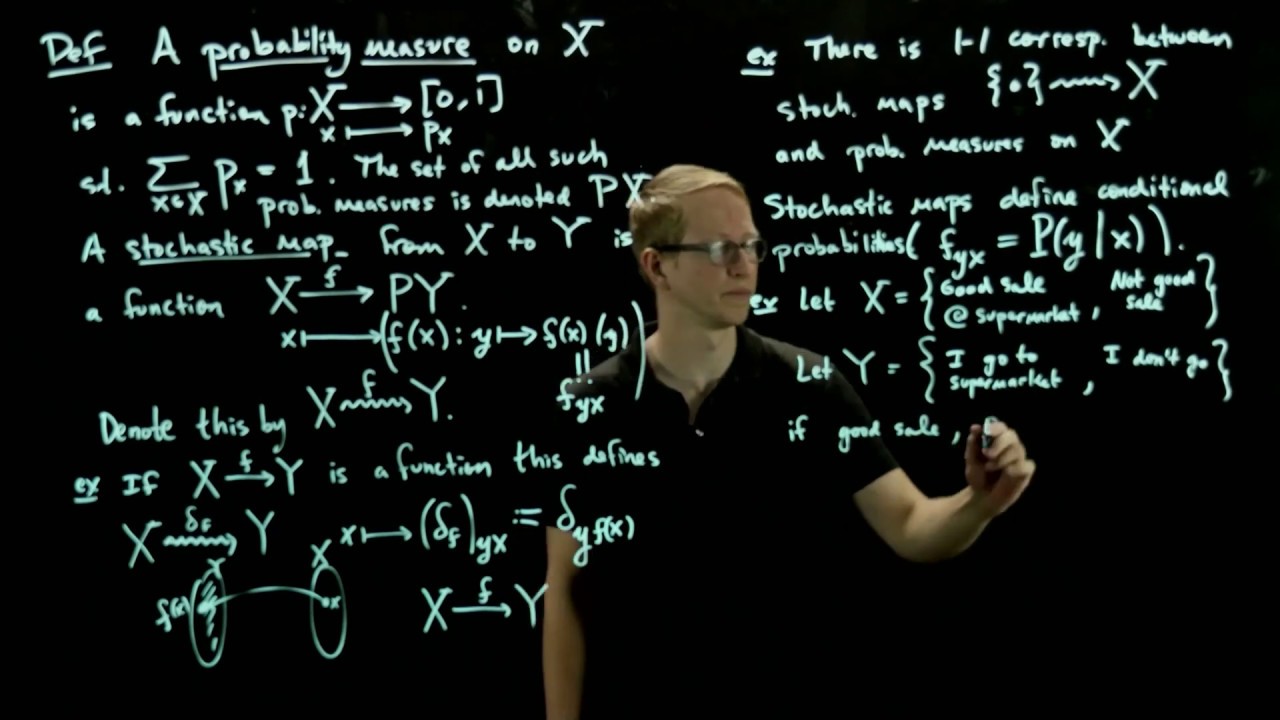
This is part of a series of lectures on special topics in linear algebra. It is assumed the viewer has taken (or is well into) a course in linear algebra. Some topics may also require additional background. Topics covered include linear regression and data analysis, ordinary linear differential equations, differential operators, function spaces, category-theoretic aspects of linear algebra, support vector machines (machine learning), Hamming's error-correcting codes, stochastic maps and Markov chains, tensor products, finite-dimensional C*-algebras, algebraic probability theory, completely positive maps, aspects of quantum information theory, and more.
These videos were created during the 2019 Spring/Summer semester at the UConn CETL Lightboard Room.
0 Comments